It doesn’t matter what you think about Macs, you have to admit that Apple’s design language is very recognisable and well-liked. The PC industry has recently responded with its own design innovations. To wit, Acer’s new Aspire S line, which consists of two different all-in-one desktop designs, is obviously aiming to steal some of the iMac’s thunder. Later in this year, you’ll be able to find them in stores.
The Aspire S will be offered in a 27-inch and a 32-inch screen size, with a subdued aluminium blue finish for the former and a kind of goldish-bronze for the latter. A single speaker sits atop the base, and all of the internal components are crammed within the column-shaped stand. Ultra-narrow borders surround the 1440p LCD screens.
The hardware’s internals are nothing to scoff at, despite its stylish exterior. The Core i5 and i7 CPUs in the 27-inch model receive Xe graphics, while the Core i7-13700 and the new Intel Arc discrete graphics in the 32-inch model have plenty of space to breathe. (The graphics processing unit it would employ was not revealed; it is likely an A-series chip like those seen in laptops.) Both desktops have Wi-Fi 6E, as well as USB-C, a dedicated Ethernet connector, and an HDMI-in connection, allowing them to function as a standard display for use with other computers or gaming consoles.
For effective online conferencing, the webcam is often the weak link in all-in-one systems. Acer has developed a solution to this problem by creating a camera with detachable modules that connect and remove using POGO pins. The camera sensor’s 1440p resolution, Windows Hello IR compatibility, and the included ring light should facilitate a polished appearance. Remove the camera if you’d want to utilise your own video recording setup.
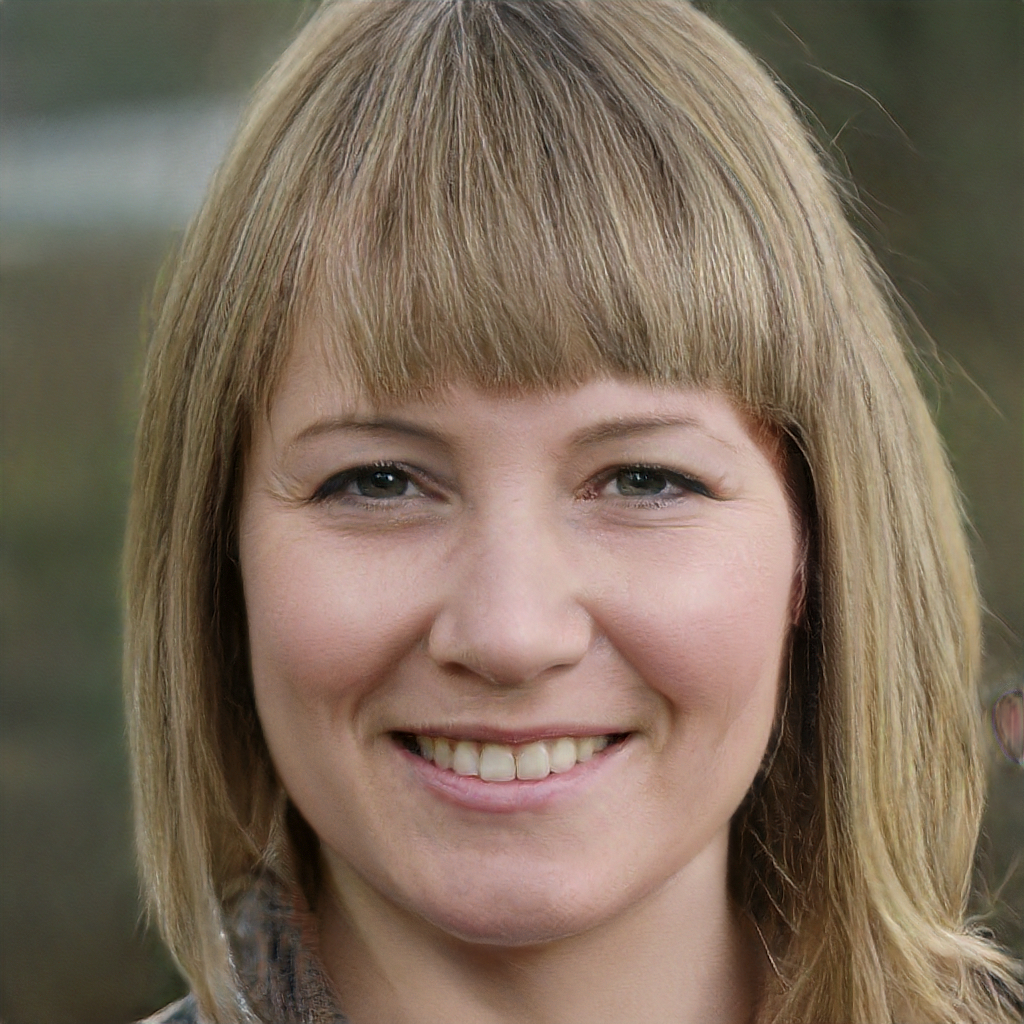
Subtly charming pop culture geek. Amateur analyst. Freelance tv buff. Coffee lover