The causes of the worker shortage in the United States have been the subject of much debate among experts. But one thing is glaringly obvious: there is a massive jobs gap of over 10 million people versus the number of people actively seeking employment (around 6 million).
In this brief article, we’ll take a step back to examine how we got here, the various causes that have contributed to this disparity, and some of the responses that have been made so far. Specifically, we’ll investigate the causes and consequences of the labour shortage in the United States, with a focus on machine learning (ML) and its applications in addressing both.
There is currently a severe shortage of workers in the United States.
The U.S. Chamber of Commerce reports that the labour force participation rate has decreased from 63.3% to 62.2% over the past few years. A 1% drop in the labour force participation rate might not seem like a big deal until you consider that it follows a pandemic that caused over 30 million people to lose their jobs.
Hospitality, food service, manufacturing of long-lasting goods, education, and healthcare are among the hardest hit sectors. Nonetheless, there is hardly any industry that hasn’t been impacted.
The shortage of workers has led to many problems, what are some of them?
The spread of COVID-19 unquestionably caused a tremor in the job market. Research indicates that about a quarter of a million people of working age perished as a direct result of the disease, another half million have left the workforce due to long-term health concerns associated with the virus, and a similar number have gone directly from illness into retirement.
This decline in employment should have been offset by new entrants to the labour force, but this has not occurred. The U.S., on the other hand, has seen a monthly increase in the quit rate across all industries. The monthly quit rate for some industries, including the leisure and hospitality sector, is above 6%. Even in seemingly safe industries like business and professional services, where turnover is usually much lower, the number is still alarmingly high at over 3%.
Numerous workers have voiced their desire to maintain their remote work arrangements. Some industries, like healthcare and manufacturing, find it challenging to meet this expectation. However, this change in perspective is only the tip of the iceberg for employees. Companies are slow to respond to the shift in employee expectations, which includes a greater emphasis on work-life balance, shorter work weeks, and ongoing opportunities for professional development and education. This helps to explain why, despite a significantly higher-than-average rate of hiring across the country, millions of jobs remain unfilled across all industries.
In other words, what is machine learning?
While the terms “artificial intelligence” (AI) and “machine learning” (ML) are often used interchangeably, ML is actually a subset or application of AI. In its simplest form, machine learning (ML) is the use of large datasets to train computers to learn something new from scratch using statistical and mathematical models.
One common use of machine learning is in image recognition. Face recognition (or “tagging” on social media) and cancer detection in x-rays are just two examples of how far image recognition technology has come.
Statistical arbitrage, which employs algorithms to analyse securities in relation to predetermined economic variables, is another common application of ML in the financial sector.
With the help of ML, computers can analyse massive datasets, discover relationships between variables, and extrapolate based on their findings. Maximizing data usage through predictive insights. This sort of foresight is used in a variety of industries, including those concerned with pricing property and creating new products. As an added bonus, predictive analytics can help both job-seekers and recruiters find more compatible candidates.
How does machine learning help alleviate the labour shortage in the United States?
Workers in the United States are having trouble finding positions that are a good fit for them, which is a major contributor to the current labour shortage and the alarmingly high quit rate.
Advanced algorithms and statistical analysis of big data are being used by both recruiters and job seekers as a means of coping with this issue.
Using ML, we can compare the characteristics and abilities of employees who voluntarily leave or are terminated from their positions to those who remain in their positions and are eventually promoted. Having this knowledge allows hiring managers to swiftly and accurately eliminate candidates who are not a good fit for the position. The end result is a more efficient job search with much better odds of success.
In addition to improving matching, ML also reduces the time and effort required for hiring. A job seeker’s mood can be made worse by the time and effort spent applying for and then interviewing for a job they are not likely to get or be happy with. We need job-seekers who are excited about the recruitment process rather than frustrated by it as we face a crisis of unfilled positions and a high quit rate.
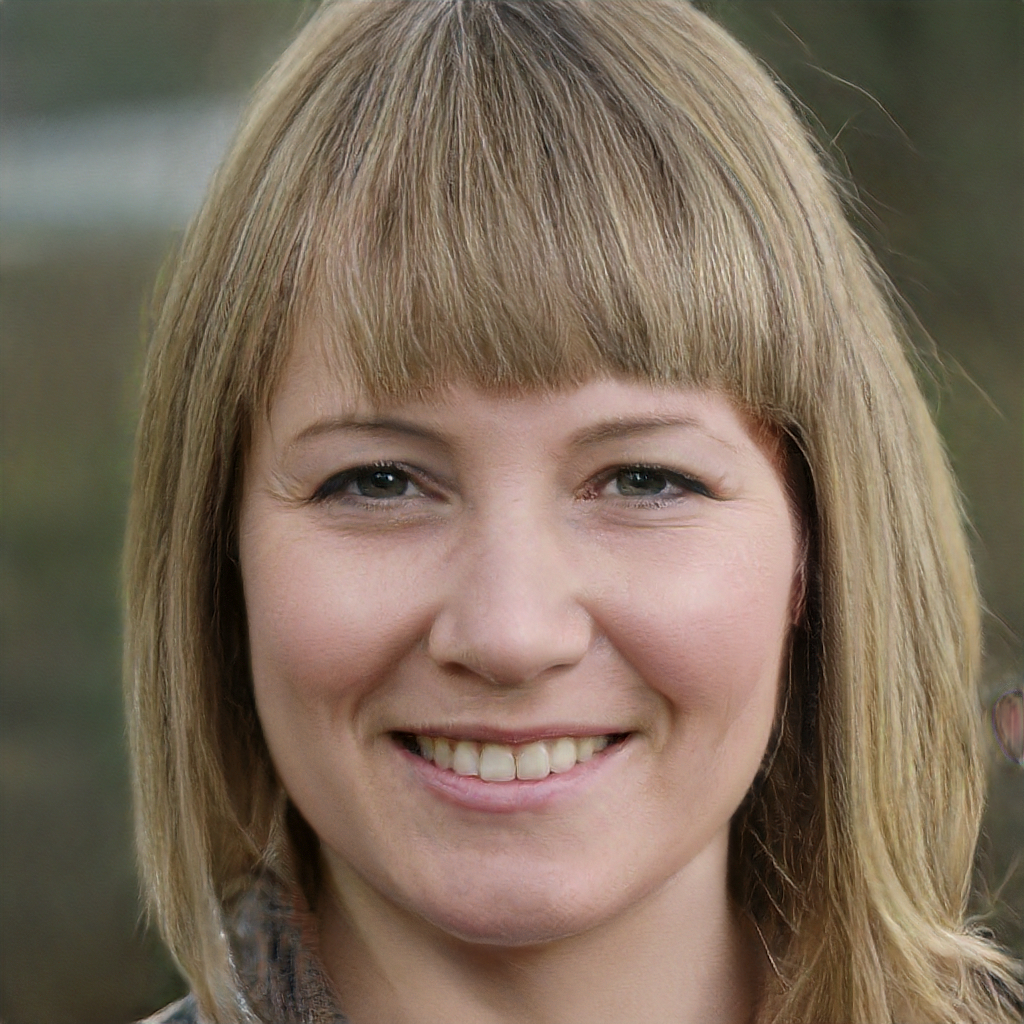
Subtly charming pop culture geek. Amateur analyst. Freelance tv buff. Coffee lover