In any organization, time series forecasting plays a key role, impacting a wide range of activities like resource planning, budgeting, and more. It’s all about predicting future trends based on past data—whether it’s sales numbers, inventory levels, or staffing changes.
What Is Time Series Forecasting, Anyway?
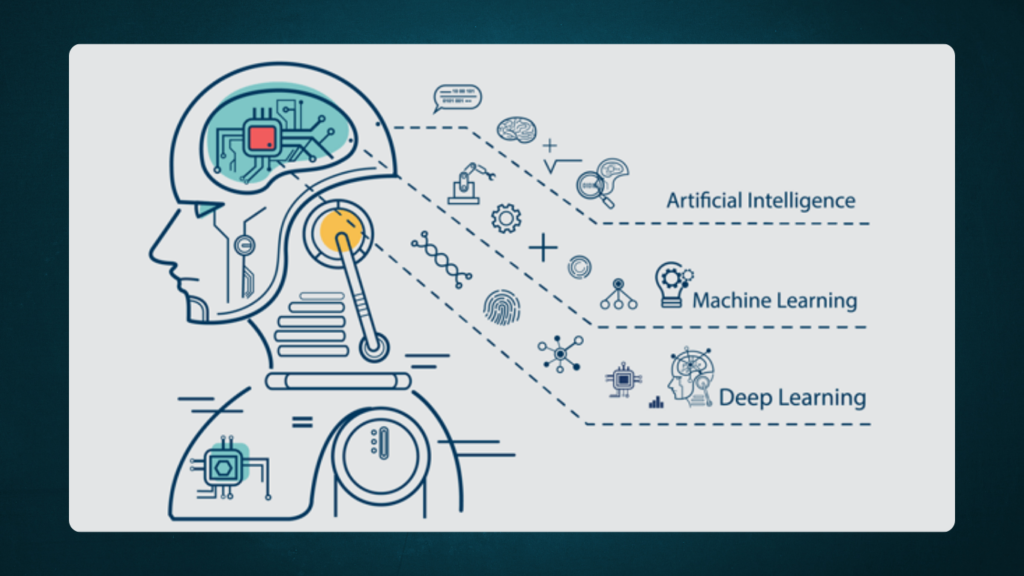
When we talk about time series, we’re referring to data points (sales, stock levels, you name it) laid out in chronological order. Time series forecasting is just a fancy way of saying we’re trying to predict what’s going to happen next, based on what’s already happened. By digging into trends, patterns, and seasonality, businesses can make educated guesses about the future—and those guesses matter.
The Role of AI in Forecasting
AI is everywhere these days, but it can mean different things to different people. In forecasting, though, machine learning (ML)—a branch of AI—really shines. ML models are great for sifting through huge amounts of data and spotting patterns we humans might miss. This ability to “see” hidden trends is what makes ML so valuable when it comes to making predictions that can shape a business’s future.
Different Approaches to Forecasting
When it comes to forecasting, there are a lot of different approaches. On one end, you’ve got simple, or “naïve,” methods that basically just assume the future will look a lot like the recent past. On the other, you have sophisticated ML models that require training, validation, and fine-tuning to give more accurate predictions.
But now, something new is shaking things up: zero-shot inference. This method doesn’t need any specific training on your data to make predictions—it’s like showing up to a test without studying for that particular subject but still scoring high because of everything you’ve learned over the years.
What’s Zero-Shot Inference?
Zero-shot inference refers to a model’s ability to handle tasks without having been trained on those specific tasks. It’s a bit like a student acing an exam based purely on their overall knowledge, even though they didn’t study that exact material. Pretty cool, right?
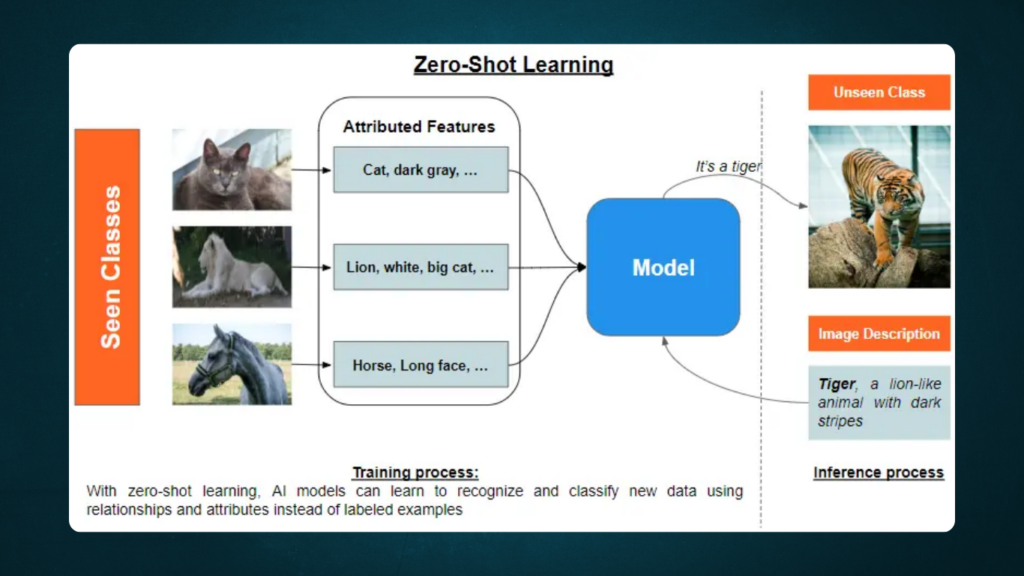
This approach has been used in natural language processing (NLP) and computer vision for a while now, but in October 2023, a company called Nixtla brought this concept to time series forecasting with their product, TimeGPT. It’s the first of its kind—a pre-trained foundation model that can make predictions based on any time series data, and it’s set to change how companies, big and small, handle forecasting.
How Does TimeGPT Work?
Foundation models like TimeGPT are trained on tons of time series data across different industries—think finance, retail, web traffic, and more. For example, TimeGPT used over 100 billion data points during its training phase. Much like the language models that power ChatGPT, these models can make predictions once they’ve been trained. The best part? They don’t need to be fine-tuned for each new dataset you throw at them. Just plug in your data, and the model does the heavy lifting.
Let’s say you provide TimeGPT with your weekly sales data for the past year. The model will use what it’s learned from other datasets to predict your sales for the next week (or whatever time period you want), all in a matter of seconds. It’s quick, easy, and you don’t have to worry about the complex processes that usually come with training traditional ML models.
TimeGPT vs. Traditional Models: The Test Drive
We ran a test using real sales data from Tielka, an Australian tea company, and compared TimeGPT to traditional models like ARIMA and Prophet. TimeGPT came out on top across the board—in accuracy, ease of implementation, and cost efficiency. While more research is needed to see how it handles longer forecast horizons and different industries, the early results are promising.
Imagine the productivity boost this could bring to your business. With better budget forecasting, you could optimize inventory, reduce costs, and ensure you have the right staff levels for peak seasons. The potential is huge!
But What About Local Needs?
Here’s where things get a bit tricky. Models like TimeGPT are often trained on data from the northern hemisphere. So while they might be great at predicting trends around, say, Thanksgiving in the U.S., they might not be as useful in Australia, where our seasons and holidays are different. It’s a reminder that while these AI models are powerful, they still need to be tailored to local contexts.
Australia’s AI Future: A Call for Action
To fully benefit from breakthroughs like TimeGPT here in Australia, we’ll need a more coordinated approach.
- Policy Framework: We need national policies on AI that cover everything from data protection to ethical standards. Without a unified approach, we risk the same fragmented responses that slowed things down during the COVID pandemic.
- Data Access: Creating national data repositories and open data initiatives will be key to ensuring models like TimeGPT can be trained on relevant Australian data. Public access to these datasets will make it easier for companies to leverage AI for their specific needs.
- Talent Development: We also need to invest in homegrown talent. By fostering partnerships between industry and academia, we can ensure there’s a steady pipeline of data scientists and engineers to keep AI innovation alive in Australia.
What Can Businesses Do Now?
If you’re running a business, there are some practical steps you can take to get ahead of the curve:
- Spread the Word: Now that you know about zero-shot inference, share it with your team. Brainstorm how new forecasting methods like TimeGPT could streamline your operations and save money.
- Hire Smart: One talented data scientist can drive more value with AI tools than a whole team of traditional forecasters. Consider investing in ML expertise within your company.
- Test and Scale: Start with pilot programs to see how these new forecasting models work for your specific needs. Once you’ve ironed out any issues, scale the solution to other areas of your business.
- Monitor Performance: Regularly check how these AI tools are performing. Keep stakeholders in the loop, and adjust as needed to ensure you’re maximizing their potential.
Looking Ahead
AI and ML are already driving massive changes across industries, and the possibilities for forecasting are just one piece of the puzzle. For Australia to remain competitive, we need to keep investing in infrastructure, talent, and the AI models that can address our unique challenges. In the end, it’s not about AI taking jobs—it’s about who can use AI to get ahead.
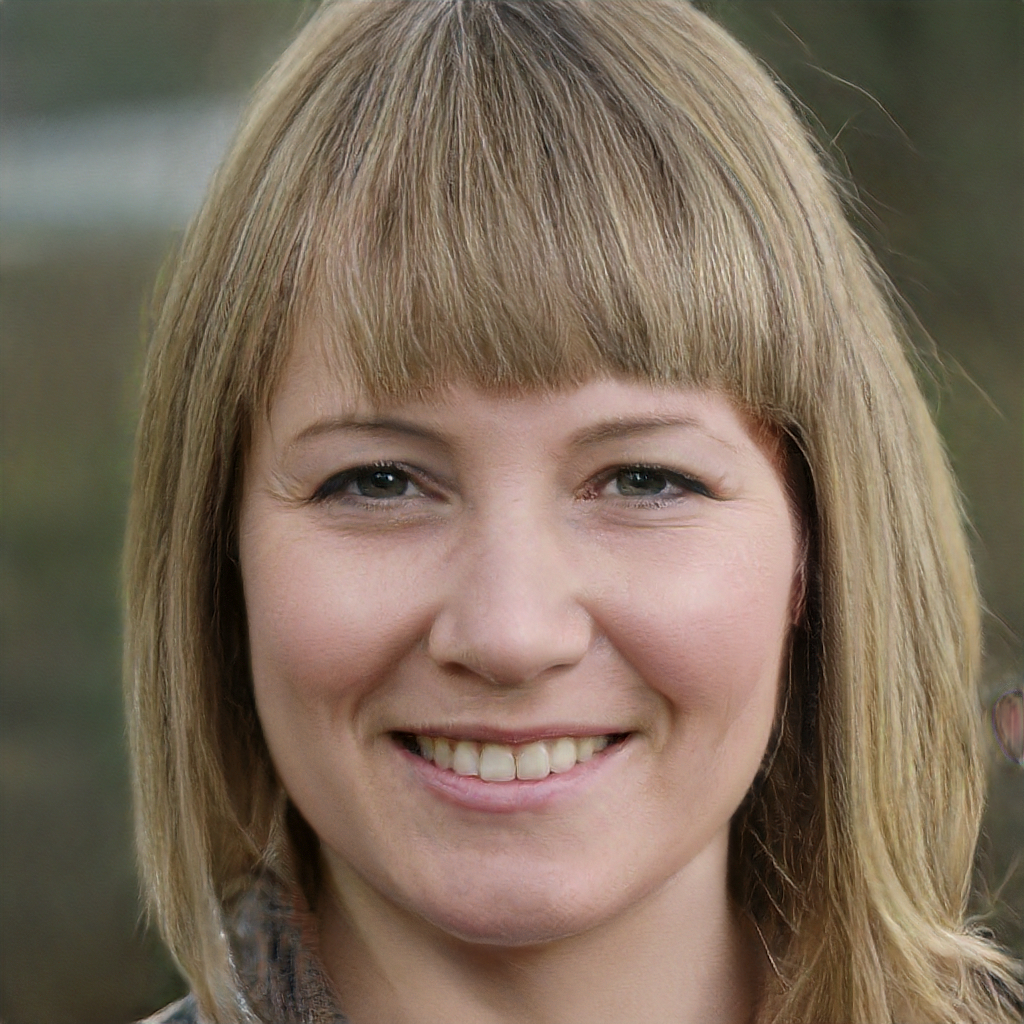
Subtly charming pop culture geek. Amateur analyst. Freelance tv buff. Coffee lover