In a recent study published in npj Science of Food, researchers unveiled VirtuousMultiTaste, a cutting-edge machine-learning tool designed to differentiate between sweet, umami, and bitter tastes based on a compound’s molecular structures and underlying physicochemical properties.
Study Overview
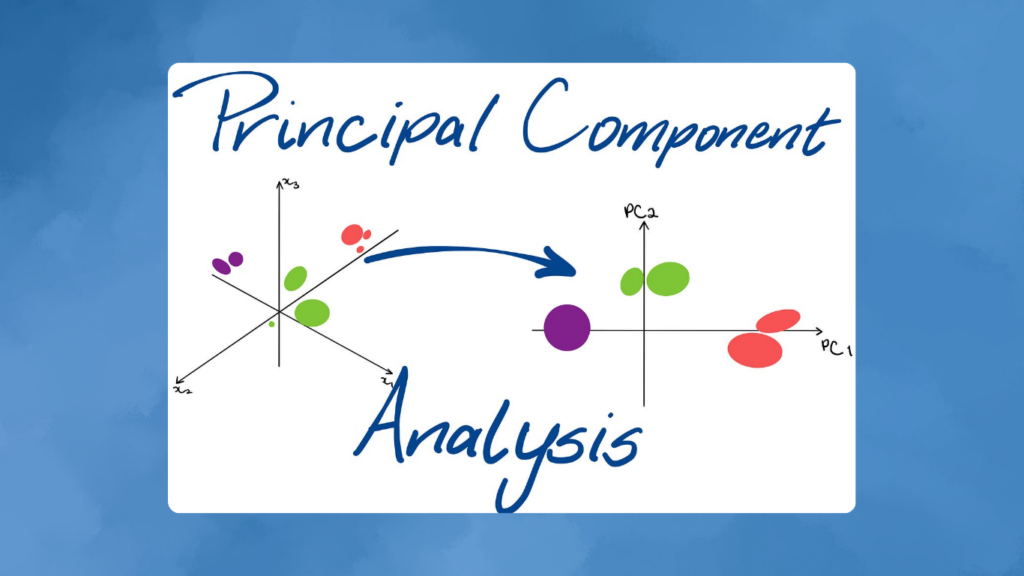
Researchers utilized machine learning and heuristic optimization techniques to predict various taste experiences in chemical compounds. The dataset included publicly available compounds with validated flavors, categorized into nine taste groups. The initial database contained 5,290 chemicals for sweet and bitter tastes and 2,549 for umami. The final dataset comprised 4,717 chemicals, with a randomly selected subset used for training. To oversample the umami class, the researchers employed the Adaptive Boosting (AdaBoost) method, adding 133 samples as an additional pre-processing step.
Principal Component Analysis (PCA) was used to evaluate molecular characteristics, identifying 1,306 features for dimensionality reduction. The most frequently used descriptor class among the 15 selected features was the Autocorrelation of a Topological Structure (ATS). Ensemble dimensionality reduction using Pareto-based optimization algorithms was applied to enhance prediction accuracy, minimize the number of selected features, and streamline classification. The optimization objectives included Accuracy (ACC), Feature Number Minimization, F1 Score, Precision (PRC), F2 Score, Recall (REC), Area Under the Receiver Operating Characteristic Curve (AUC), Manhattan Distance, and Tree Number Minimization.
Random forest (RF) classifiers outperformed support vector machines (SVM) across different objectives. The researchers compared 20 different RF models, selecting the best-performing one based on performance and minimal feature set. They utilized 10-fold cross-validation (CV) on the training dataset. Autocorrelation descriptors were computed using Moreau-Broto autocorrelations weighted by various properties, including Allred-Rocow, Pauling, and Sanderson electronegativity, mass, Gasteiger charge, atomic number, ionization potential, polarizability, and intrinsic state.
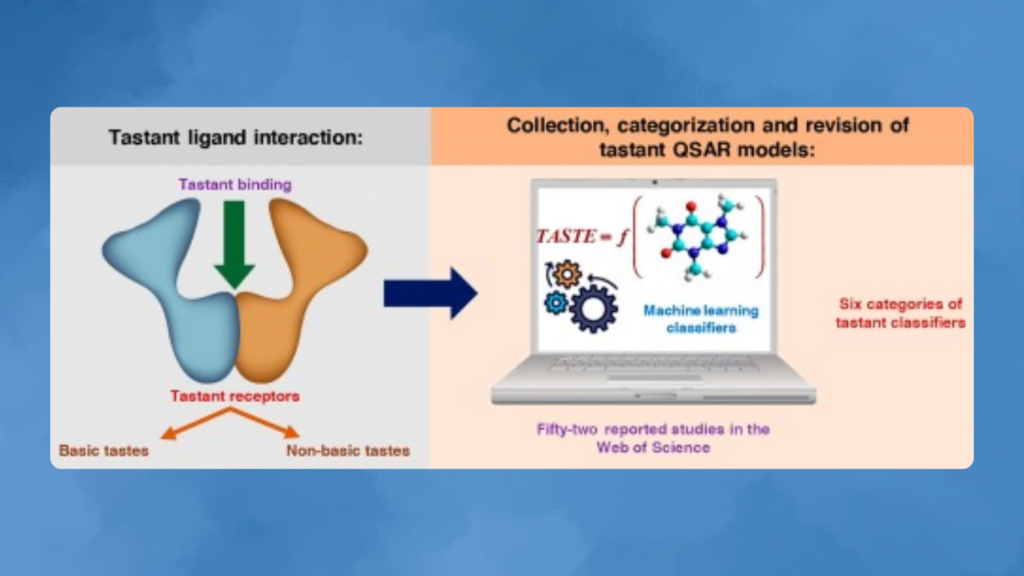
The model’s performance was evaluated against external food and natural product databases such as FooDB, FlavorDB, PhenolExplorer, Natural Product Atlas, and PhytoHub. Coffee and chocolate were analyzed based on their proportional content in FooDB. Additionally, the model was compared with commonly used machine learning algorithms and pipelines.
To assess model applicability, the researchers compared the similarity between tested substances and chemicals used during training using Morgan Fingerprints and the Tanimoto Similarity Index. The VirtuousMultiTaste tool was also compared with previously developed VirtuousBitterSweet and VirtuousUmami taste predictors.
Background
Taste and smell are essential for food chemosensory perception, playing a significant role in meal selection and consumption. Taste perception relies on five primary sensations: sweet, bitter, umami, salty, and sour, which help regulate nutrient intake and guard against harmful substances. Understanding the physicochemical properties of food components is crucial for influencing flavor perception and consumption habits.
While previous machine learning-based algorithms have classified the tastes of chemical compounds, there is still room for improvement in developing multi-class models capable of predicting the full spectrum of fundamental tastes. This limitation poses challenges for advancements in food science and technology.
Results
In cross-validation, the selected RF model achieved an AUC value of 0.92, 77% accuracy, and 77% recall. The test set showed an AUC of 0.87, with 79% accuracy and 72% recall. The umami flavor had the highest AUC value (0.98), followed by the bitter taste (0.92) and the ‘other’ taste group (0.86). The VirtuousMultiTaste model demonstrated superior performance in predicting bitter taste, with accuracy, precision, recall, and F1 and F2 values of approximately 83%. Coffee exhibited a predominantly bitter taste profile, with 130 predicted bitter chemicals, while chocolate contained 96 bitter compounds, 33 sweet compounds, four umami compounds, and 13 other taste compounds.
VirtuousMultiTaste outperformed other classifiers in performance metrics. VirtuousMultiTaste and VirtuousUmami had comparable accuracy and AUC ratings but showed slightly lower precision, recall, F1, and F2 values. Both methods achieved over 99% accuracy in evaluations using non-umami chemicals not used in training. VirtuousMultiTaste could predict umami compounds beyond peptides, allowing for a broader chemical analysis. Model performance remained consistent across similarity quartiles, indicating widespread applicability.
The VirtuousMultiTaste tool can rapidly analyze chemical databases for candidate compounds with predicted taste qualities. It has shown exceptional capability in predicting multiple taste sensations concurrently, suggesting its potential integration into multisensory perception studies. The tool predicts four tastes and facilitates the analysis of various chemicals and understanding of the chemical-physical processes influencing overall taste perception.
However, interpreting the chemical and physical properties of tastants based on 15 primary characteristics remains challenging. Future research should focus on developing simpler descriptors or specific methods for correlating molecular descriptors to structural characteristics or functional groupings.
Conclusion
The VirtuousMultiTaste machine-learning tool represents a significant advancement in taste prediction technology, offering a powerful method for analyzing the taste qualities of chemical compounds. Its ability to predict multiple taste sensations with high accuracy and applicability across various chemical datasets holds promise for future developments in food science and technology.
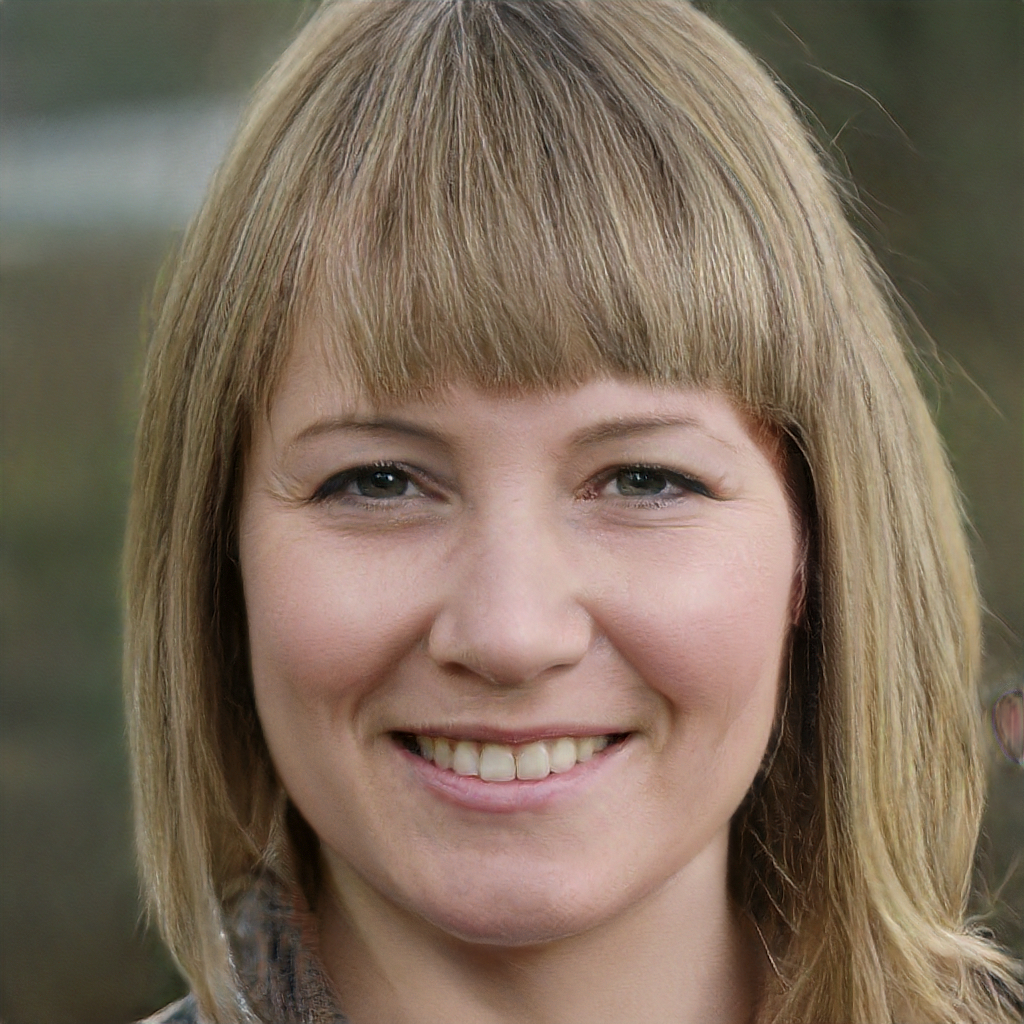
Subtly charming pop culture geek. Amateur analyst. Freelance tv buff. Coffee lover